[LUM#22] Concrete predictions
Reactor containment, cooling towers, spent fuel storage pools... in a nuclear power plant, concrete is omnipresent. And it's subject to numerous stresses: radiological, thermal, chemical, hydric and mechanical. To predict the watertightness of these installations, Yann Monerie, researcher at the Mechanics and Civil Engineering Laboratorylaboratory, uses artificial intelligence.
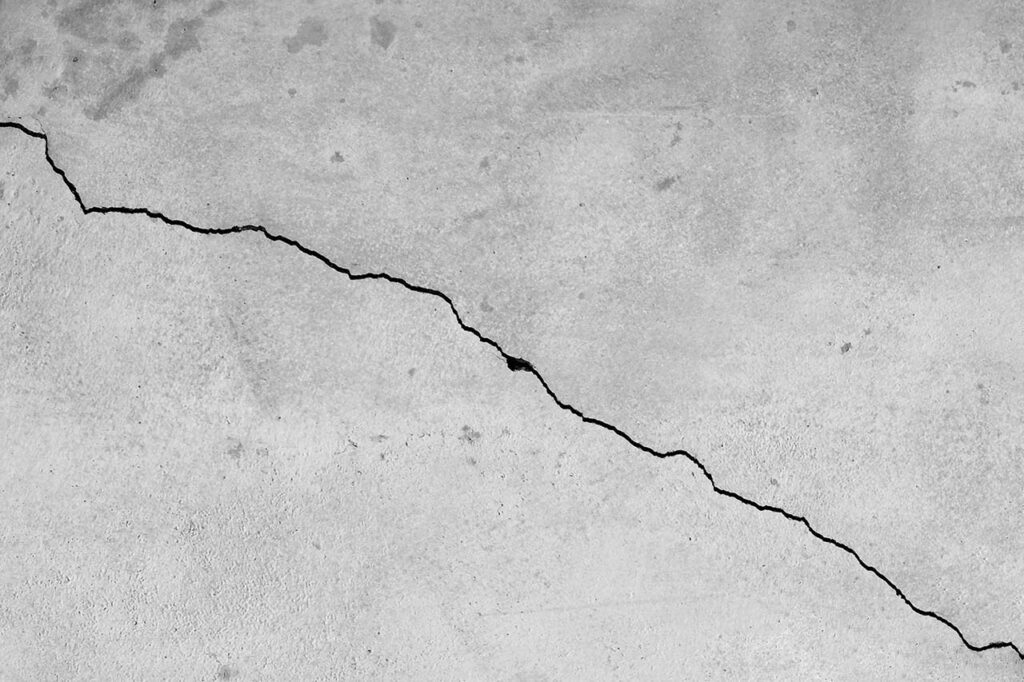
Over 20 million tonnes of concrete are produced in France every year, to build buildings, dams, bridges and even... nuclear power plants. A high-risk installation where this mixture of sand, cement, gravel and water acts as a containment barrier. But how do we know that this enclosure will remain watertight over time? "The watertightness of concrete is determined by the way it cracks. The more tortuous the path of these cracks, the less easily fluids flow through, and therefore the tighter it is," explains LMGC researcher Yann Monerie.1.
Tortuosity
To determine this tortuosity, you first need to know the properties of the various components in the mix, but also how the aggregates are distributed in the mortar. " Concrete is not homogeneous, and the spatial distribution of its different phases determines its cracking," explains the specialist in micromechanics of materials, who is collaborating on this issue with the French Institute for Radiation Protection and Nuclear Safety.
Because cracks don't propagate randomly, but evolve from one gravel to another. " The interfaces between the aggregates and the matrix are the weak zones that determine crack propagation". It is this trajectory that the researchers want to predict in order to better understand the mechanical properties of this material.
From 4 months to 3 seconds
And to make these predictions, Yann Monerie and his colleagues are using artificial intelligence. " We have developed a model that enables statistical reconstruction of the three-dimensional microstructure from two-dimensional sections," explains the researcher.
After studying thousands of photos of concrete sections and their 3D correspondence, AI enables them to deduce from simple images the size and shape of aggregates, as well as how they are distributed in the cement. This modeling enables the researchers to establish a numerical simulation of crack propagation. "Without machine learning it takes around 4 months of calculations to carry out these simulations, with AI it takes 2 to 3 seconds!"
This predictive artificial intelligence makes it possible to predict the permeability of a power plant based on simple cross-sectional drawings of concrete samples. And these applications extend even beyond the nuclear sector: "With this method, we can also determine the watertightness of houses built on polluted soil, for example", adds Yann Monerie.
UM podcasts are now available on your favorite platforms (Spotify, Deezer, Apple podcasts, Amazon Music...).
- LMGC (CNRS, UM)
︎