Soccer: using data to improve performance
Our society is becoming increasingly digitized, thanks to the use of a wide range of digital technologies that generate large flows of data grouped under the term Big Data. This is particularly true of sports-related data collected via connected watches, smartphone applications, satellite tracking systems and smart textiles.
Stéphane Perrey, University of MontpellierGérard Dray, IMT Mines Alès - Institut Mines-Télécom and Jacky Montmain, IMT Mines Alès - Institut Mines-Télécom
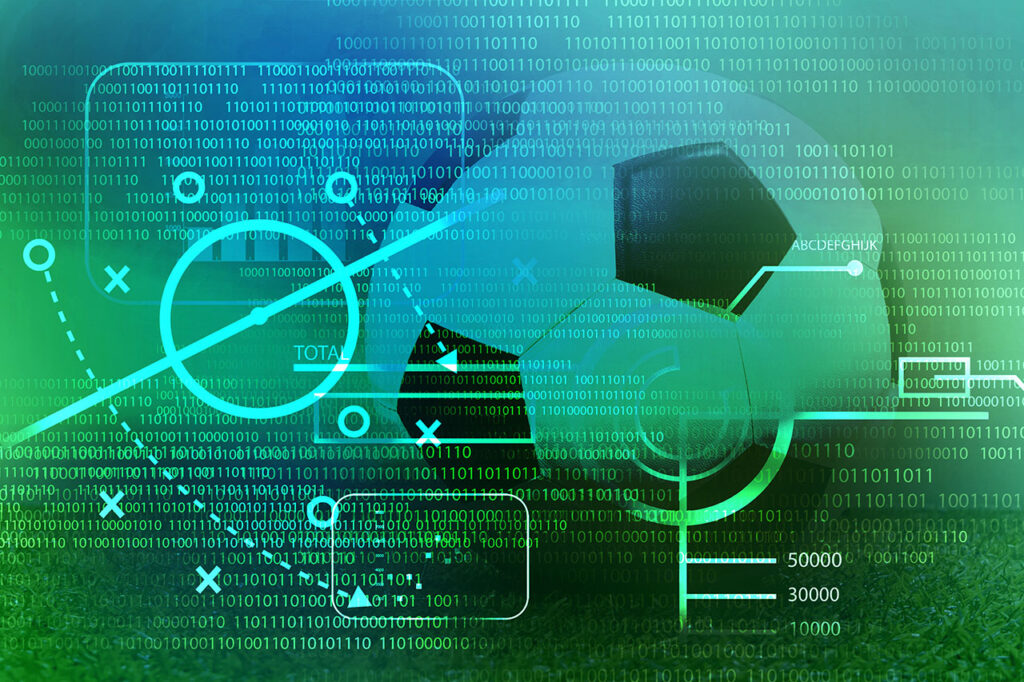
This data would enable us to identify key performance indicators, as well as the movements of athletes on the field: number and size of sprints and changes of direction, distance covered at certain speeds, changes in heart rate or player trajectory.
They can thus guide training strategy. It's only a short step from there to becoming an essential tool for improving performance or preventing injury. This type of information can therefore be of interest to a wide range of players: athletes, coaches, physical trainers, adapted physical activity teachers, doctors and sports agents. These data, and the methodologies that accompany them, open up new prospects for research in sports science and digital science.
Recent developments in wearable and connected sensors, cloud-based data storage and artificial intelligence tools have been the pillars of a major shift in the way sport-related data is analyzed. Over the last decade, sports science research has benefited from the reduction in sensor size and the consequent increase in the ability to collect and then analyze simultaneous measurements, driven by huge advances in wireless transmission.
Soccer is one of the most popular sports in the world, and over the years more and more information has become available, increasing the interest of data analysts in the sport. The associated professions, data scientist, have developed with specific skills in statistics and IT in order to collect, process, analyze and make massive data talk... with the aim of finding useful information for decision-making.
In the world of sport, the term "Sports Data Analyst" has been coined, and several think tanks, university schools and thematic research units(EuroMov Digital Health in Motion) have been set up, bringing together multi-disciplinary expertise. How can sport science and digital science, from data collection to data management and predictive modeling of performance or injury, impact the field of sport?
The proliferation of movement data
Recent years have seen the rise of position detection systems to provide spatio-temporal tracking data for players on the pitch. Although semi-automatic camera systems have been used to track the position of players at professional soccer matches, automatic tracking systems using satellite positioning systems (GNSS for Geolocation and Navigation Satellite System) or local positioning measurement systems are commonly adopted by professional structures and teams in team sports (soccer, rugby, basketball, handball, ice hockey). Some positioning systems have even fitted balls with integrated sensors.
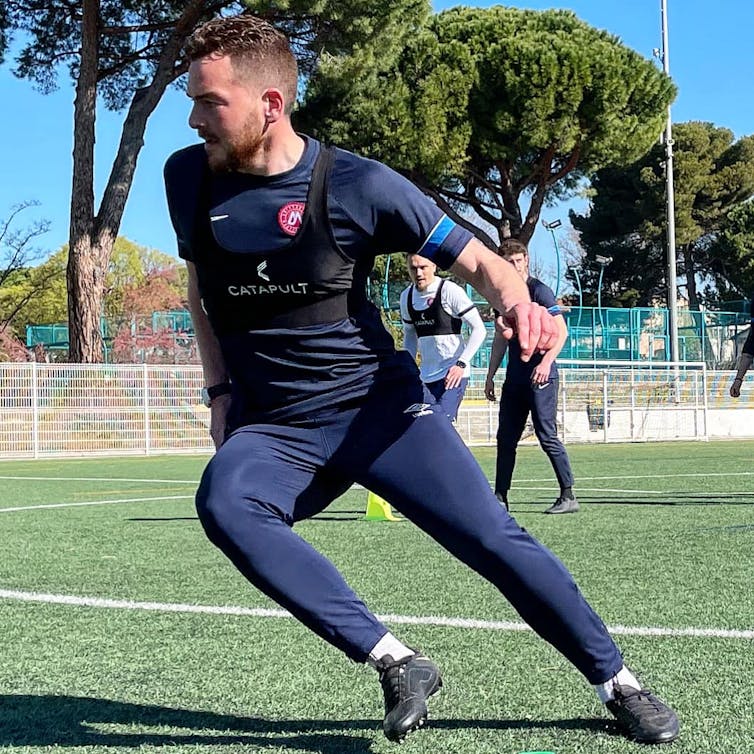
One of the most visible advances in many sporting disciplines has been the introduction of autonomous inertial measurement units (IMUs) to measure linear accelerations (accelerometers), rotational speeds (gyroscopes) and the earth's magnetic field to orientate the unit (magnetometers) of a point in three dimensions.
The fusion of these portable sensors (IMU-GNSS) is a constant trend in the development of systems for tracking and detecting human movement, with applications in sports such as soccer for tracking players' motor actions. Generally speaking, displacements (speed, distance and derived measurements) are quantified primarily from GNSS data, while shock/impact detection and characterization is reserved for IMU data. Despite these advances, is it possible to free the player from these sensors in motion quantification? It would seem so.
[Nearly 80,000 readers trust The Conversation newsletter to better understand the world's major issues. Subscribe today]
Markerless motion capture systems and algorithms have been constantly improved over the last five years to measure kinematics (i.e. the description of movement in terms of position, velocity or acceleration) in sport. Modern computer vision algorithms using neural networks have been adapted to assess different forms of motor action, providing practical means for faster data analysis with validity in real-life conditions, i.e. outside the restrictive environment of the laboratory.
The classification of human locomotor activity typologies from a sports performance perspective can be enhanced when acquired signals are used as inputs for machine learning algorithms. The ability of current algorithms to analyze and extract knowledge from such datasets can, for example, identify changes of direction, often decisive in matches. The use of deep learning algorithms (a sub-field of machine learning concerned with algorithms inspired by the structure and functioning of the brain, known as artificial neural networks) on video images, would enable us to recognize different types of soccer strike with great precision.
Using several IMU-GNSS fused sensors and different machine learning algorithms, Reilly et al. developed an automated classification model to accurately identify player movements with changes in direction during competitive matches. Improvements in classification models in sports such as soccer are always challenged by the variability of movement patterns, which has a direct impact on the quality and quantity of available datasets.
From data to prediction of performance or injury states
So how can Big Data enhance performance in top-level sport? The data collected by the aforementioned tools and equipment can be used to characterize in detail the movements produced, and then to determine the athlete's physical training dose. Widely used to monitor soccer players, physical demand can be determined using objective mechanical parameters, calculated from GNSS-IMU signals coupled with heart rate signals, for example. The data collected from these wearable devices provides useful information for understanding a player's activity, his performance in competition or preventing the risk of injury during training.
One approach is to carry out descriptive analyses, so as to characterize target exercise intensities in relation to physical performance over time, and bring out interpretable analytical relationships. With sufficient information gathered over several months or even years, predictive analyses can be deployed to estimate performance on "D" day, or provide useful information for coaches, teams or players to guide training protocols to optimize prescription (volume, intensity and type of exercise) and thus performance.
With regard to individual physical performance in professional footballers, researchers have presented an approach that predicts individual acceleration-velocity profiles from GNSS data measurements in real match situations. These profiles can provide relevant information concerning the theoretical maximum strength of hip extensors and the ability to produce significant horizontal force at high running speeds; these factors being key determinants of the onset of muscular injuries, or sprint performance.
In the context of sports injuries, the ability to predict risk factors and assess an athlete's state of readiness after surgery or any other intervention is essential. The application of machine learning techniques appears to be able to provide information on the risk of non-contact injuries by taking into account changes in training doses over one week (short term) and one month (medium term) based on data collected by GNSS and IMU coupled with questionnaires. The results of a study carried out over the course of a sports season on professional soccer players in Ligue 2 showed that, depending on the complexity of the predictive model, classification performance in predicting injury risk can approach 100%, particularly over a one-month time horizon.
In addition, it appears that subjective variables (such as sleep quality, fitness, mood, satisfaction and enjoyment) are important factors in predicting injury risk, as is distance covered. This initial information can be used to guide individualized training programming to reduce the risk of injury.
Today, wearable mobile devices provide the information needed to analyze player performance in training and competition. What's more, new machine learning algorithms, notably deep learning and data mining, enable players' progress to be assessed in all phases of their training.
However, it remains to be seen whether current technological and scientific advances are already mature enough to materialize live automated decision-making systems in real competition conditions and influence the outcome of the match.
Stéphane Perrey, University Professor in Exercise Physiology / Integrative Neuroscience, Director of the Research EuroMov Digital Health in Motion Unit, University of MontpellierGérard Dray, Professor, IMT Mines Alès - Institut Mines-Télécom and Jacky Montmain, Professor - EuroMov Digital Health in Motion, IMT Mines Alès - Institut Mines-Télécom
This article is republished from The Conversation under a Creative Commons license. Read theoriginal article.