[LUM#22] No AI without humans
Computers capable of learning are no longer science fiction, they're machine learning. And to learn, machines need humans to provide them with colossal quantities of data. To ensure their quality, statistician Joseph Salmon is banking on cooperation.
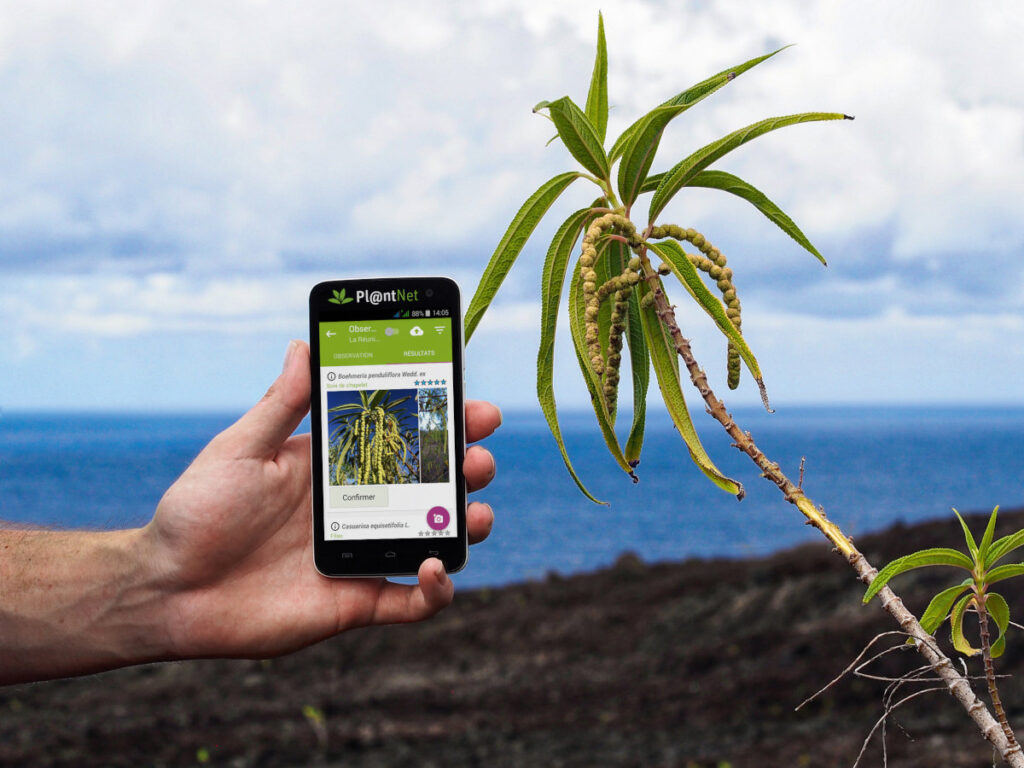
How can autonomous cars read road signs? How can a smartphone recognize the song of a nightingale or the leaf of an oak tree? If all this is now possible and even commonplace, it's thanks to machine learning. " It's the very basis of artificial intelligence," explains Joseph Salmon, a statistics researcher at the Alexander Grothendieck Institute in Montpellier, France.1.
To be able to tell the difference between a nightingale and a titmouse, or an oak tree and a poplar, the computer had to "look" at a lot of tree leaves or "listen" to a lot of birdsong. These images and sounds are data, the lifeblood of AI. "Machine learning requires large amounts of data to function properly, especially in classification tasks."
And the quality of this information, known as input data, is crucial to the success of the tasks at hand. " Garbage in, garbage out", sums up the specialist. Garbage in, garbage out. In other words, providing the computer with faulty or nonsensical data will result in equally nonsensical responses from it. To illustrate this computer scientist's aphorism, Joseph Salmon cites the well-known example of Barack Obama being recognized by an AI as a monkey. The reason: the database used by the recognition algorithms contains mostly white faces.
No AI without cooperation
So to make sure he has plenty of quality data, Joseph Salmon is banking on cooperation. In 2019, he was awarded an ANR-funded chair in artificial intelligence research and teaching. With Camelot, as it is called, the researcher and his collaborators hope to meet the challenges of identifying biodiversity through participatory science and crowd-sourcing, in other words, calling on the general public to obtain data.
A strategy already put to good use in developing the Pl@ntNet recognition application, a citizen science project designed to automatically identify plants using photos, in which Joseph Salmon was involved. "Pl@ntNet involves 25 million users providing photo data and sending back plant names. Crowdsourcing makes it possible to synthesize and use all this knowledge", stresses the statistician for whom "there is no AI without cooperation". Moreover, for the researcher, artificial intelligence is not at all artificial. "We take all the energy of human beings that we've synchronized, and the AI just acts as the link in a collective effort.
Neural network
In fact, learning to learn is nothing other than what the human brain does. To lend this power to machines, the mathematicians and statisticians working on these issues draw their inspiration from the way the brain works, in order to mimic the learning process. "The mathematical tool we use is called a neural network. Dozens or even hundreds of layers of neurons can be combined, each receiving and interpreting information from the previous layer. This is known as deep learning," explains Joseph Salmon. Mathematics is used here to create an algorithm designed to minimize error. " The neural network is a mathematical function that starts with an image at the input and has to give a name to the output, which requires huge functions to be programmed," explains Joseph Salmon.
Character recognition
In the late 90s, the first major application of deep learning was the automated recognition of bank cheques. The principle is simple: the computer must automatically recognize the amount, handwritten in figures and letters, on each cheque. The work involved is complex, however, as handwritten numbers will never be exactly the same. "To get a computer to recognize a handwritten number, we had to provide it with a large number of annotated images. In this learning game, the computer has to give the right answer while minimizing errors", explains the statistician.
During the learning process, the algorithm aims to narrow the gap between the results obtained and those expected, in order to refine its recognition. This is similar to the way the brain analyzes a multitude of imprecise pieces of information and interprets their combination to recognize a 2 as a 2, or an oak leaf as an oak leaf.
UM podcasts are now available on your favorite platforms (Spotify, Deezer, Apple podcasts, Amazon Music...).
- Imag (UM, CNRS, Inria)
︎