[LUM#22] In search of lost glaciers
Geologists use satellite images to study glaciers. Isabelle Rocamora proposes to simplify this long and tedious task by developing the very first deep learning model dedicated to the mapping of glacial moraines.
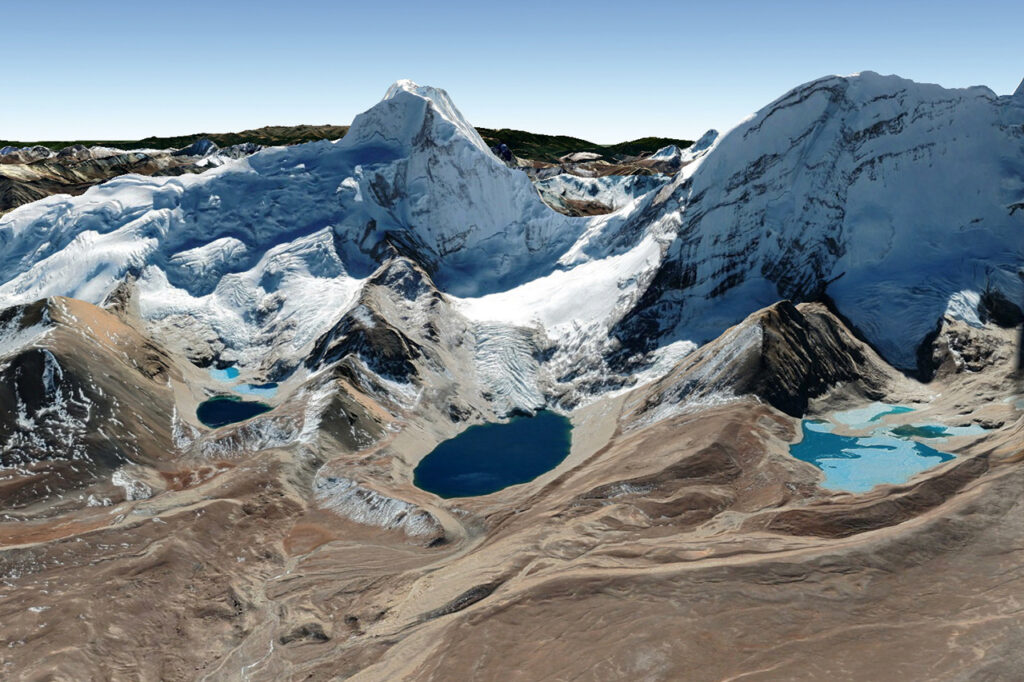
Most of the freshwater on our planet's surface is not found in rivers or lakes, but in glaciers. Although there are still almost 200,000 of them, many have disappeared and those that remain are melting like snow in the sun. Over the course of the 20th century, the glacier surface has shrunk by 30-40% in the Alps, 75% in the Pyrenees, and even more than 80% in certain tropical massifs such as the Andes or Kilimanjaro.
As the glaciers retreat, they leave behind a trace of their passage: geological forms known as moraines. It's a pile of rocky debris dragged along by the sliding movement of the glaciers and which accumulates at the bottom and on the sides," explains Isabelle Rocamora. Just like when you push sand in with your hand and pull it out, it leaves beads of sand in front and on the edges", says the doctoral student who is leading interdisciplinary work between the Geosciences Montpellier laboratory and the University of Montpellier.1 and Tetis laboratories, under the direction of Matthieu Ferry and Dino Ienco. Mapping these moraines provides valuable information, particularly on glacier retreat.
Automate tasks
This is a necessary but particularly time-consuming task: "To establish this map, we take measurements in the field and then look for the presence of glacial moraines on satellite images, which give a broader view", explains the geologist. To save time, she proposes to automate this task using artificial intelligence. "I've developed an algorithm that can analyze satellite images, it's the first deep learning model dedicated to glacial moraine mapping."
To teach her MorNet software program to recognize a moraine from another rock formation, Isabelle Rocamora began by mapping "the old-fashioned way" using satellite images of the Himalayas acquired by the CNES Pleiades satellite. "I then fed these maps into the software, telling it 'this is a moraine' or 'this is not a moraine'." The model then determined the common features between the samples so that it could learn on its own to identify a moraine on satellite images. " A computer doesn't 'see' things the way a human does, so rather than give it human answers, we're going to let it find its own identification rules," explains Isabelle Rocamora.
Contextual information
To measure MorNet's performance, the geologist then compared her own mapping with that produced by the artificial intelligence. While MorNet was effective at recognizing moraine crests, it was less effective at identifying moraine flanks, meaning that the model knew where these geomorphological formations were located, but wasn't very good at delineating them. And with good reason: a moraine is defined not just by its shape, but also by a formation pattern, and MorNet can't see this, so to improve it we'd have to give it contextual information. " Deep learning may save time, but mapping still requires the eye of a geomorphologist to confirm and refine the machine's conclusions", concludes Isabelle Rocamora.
UM podcasts are now available on your favorite platforms (Spotify, Deezer, Apple podcasts, Amazon Music...).
- GM (UM, CNRS, U Antilles)
︎